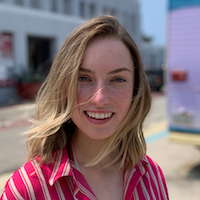
I am a Senior Research Fellow at the
Gatsby Computational Neuroscience Unit &
Sainsbury Wellcome Centre
at University College London (UCL).
Research summary: I study learning in minds, brains, and machines using a combination of behavioral experiments, computational simulations, and analytical techniques. See my research page for more.
Here is a more formal bio that can be used to introduce me.
Recent News
I’m helping to organize this year’s Conference on Cognitive Computational Neuroscience (CCN) in Amsterdam as part of the Technical Program Committee (Program Chair equivalent). The deadline for the new Proceedings track was February 20th, 2025, and we’re very excited to have received over 100 submissions!
I will give a lecture + tutorial session at the July Cognition Academy of the Max Planck School of Cognition, hosted at the beautiful Schloss Eckberg.
I’ll visit Italy to give an invited talk at Bocconi University in Milan and then head to Trieste do the same at YiHD 2025 at ICTP!
I’ll attend RLDM in Dublin to give an invited talk at the Workshop on “Representational alignment and aging.”
🧠💭🤖 Our workshop on Representational Alignment is back at ICLR 2025! Stay tuned for details of our hackathon.
I attended the Simons Institute’s Workshop on LLMs, cognitive science, linguistics, and neuroscience in Berkeley.
The 3rd edition of the Summer School on Analytical Connectionism is taking place in London this summer! If you’re a student or postdoc interested in developing and analyzing connectionist models of cognition, please apply.
I gave an invited talk at the UniReps Workshop at NeurIPS.
Leon’s paper on an analytical model of neural receptive fields was accepted to NeurIPS as a spotlight presentation! 🎉
I am serving as a DEI Chair again at ICLR 2025, this year alongside Pablo Samuel Castro.
Recent Publications
For a complete list, see my research page or my CV.
In ICLR Blog Posts Track, 2025.
To appear at ICLR 2025 as a main conference poster..
In submission, 2025.
In Advances in Neural Information Processing Systems (NeurIPS), 2024.
Spotlight presentation (<4% of submissions).
In submission, 2024.